グラフ信号処理 Graph signal processing
センサネットワーク,社会的ネットワーク,脳ネットワーク等の複雑な構造を持つネットワーク上に存在するデータを解析するために必要な信号処理・機械学習技術に関する研究を行っています.
We aim to develop signal processing and machine learning techniques for analyzing data existing on complex-structured networks, such as sensor networks, social networks, and brain networks.
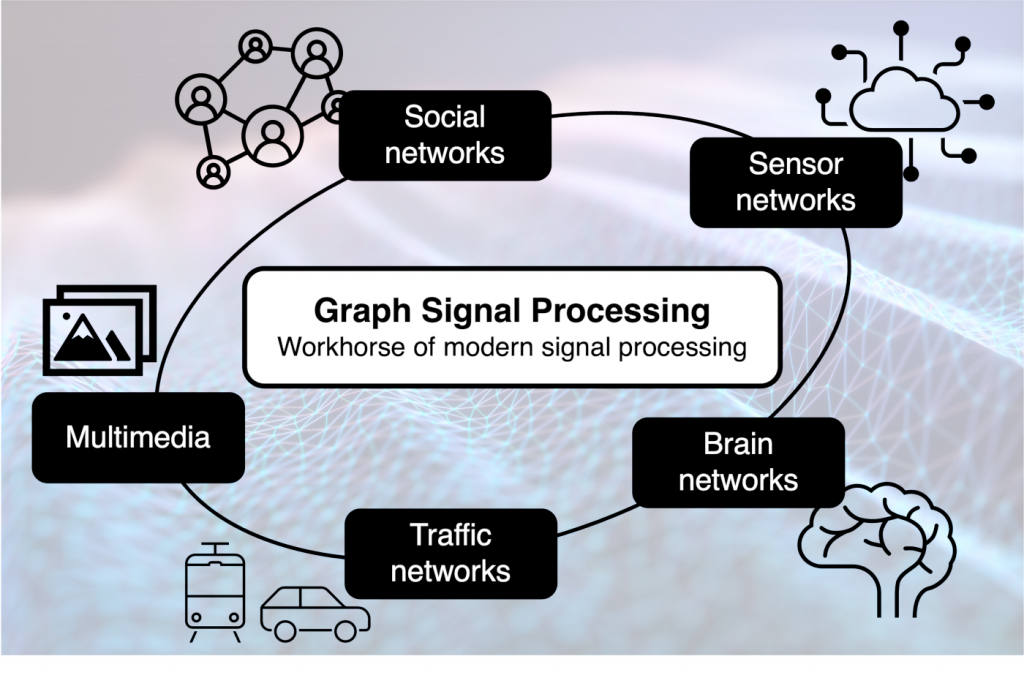
生体・神経・行動データの解析・モデル化と工学的応用 Signal processing for bio-signals, neural signals, behavioural data
生物から計測された生体,神経,行動データから,信号処理・機械学習を用いて,意味のある情報を抽出する研究を行っています.特に脳波を扱った研究では,「考えるだけ」でコンピュータの操作を可能にするブレイン・コンピュータ・インタフェースへの応用を研究しています.また,行動データを用いて,人や動物の行動(意思決定)を模倣する人工知能を作ることで,生物の行動原理の理解を目指します.これらの研究は,人に寄り添った情報通信技術の基盤となります.
We study signal processing techniques to extract desired information from bio-signals, neural signals, and behavioural data. Our goal includes brain-computer interfaces that allow us to control computers just by “thinking”. In addition, the replication of human behaviour by artificial neural networks reveals a deeper understanding of our decision-making process.
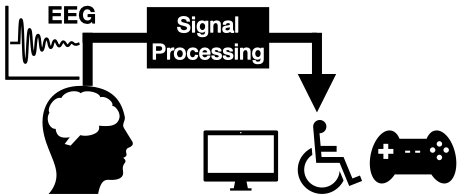
多様なセンサに対応した超スマートセンシング技術の開発 Hyper smart sensing
汎用センサ(IoTセンサを含む)から取得される多種多様なビッグデータに対して,効率的な記録・解析を実現するためのデータ圧縮やサンプリングに関する研究に取り組んでいます.センシングは,ノイズ除去,予測制御,圧縮,サンプリング,復元といった複合的な研究分野であり,その応用はAI×IoT(AIoT),自動運転,スマートシティ,医療・ヘルスケアなど多岐にわたります.優れたセンシングがあってこそ, AIや機械学習も効果を発揮します.数理的合理性と実用的価値を融合させた「超スマート」な研究を通じて,日本の未来を支える基盤技術の確立を目指しています.
We investigate data compression and sampling methodologies for efficient storage and analysis of large-scale sensor data, including those from the Internet of Things (IoT). Sensing is inherently multifaceted, encompassing noise reduction, predictive control, compression, sampling, and reconstruction. These technologies find broad application in AI×IoT (AIoT), autonomous driving, smart cities, and healthcare. High-fidelity sensing is crucial for the effective deployment of artificial intelligence and machine learning in these domains. Our “hyper-smart” research integrates mathematical rigor with practical value, aiming to establish foundational technologies that will support our future.
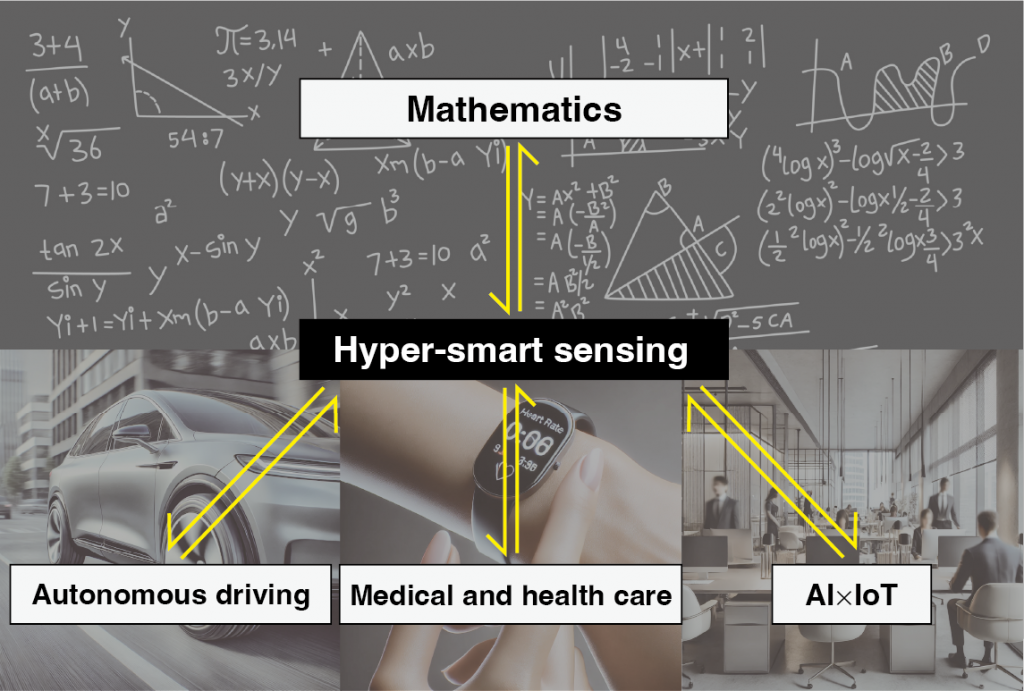